Fast AI Inference: Etched - Sohu Chip
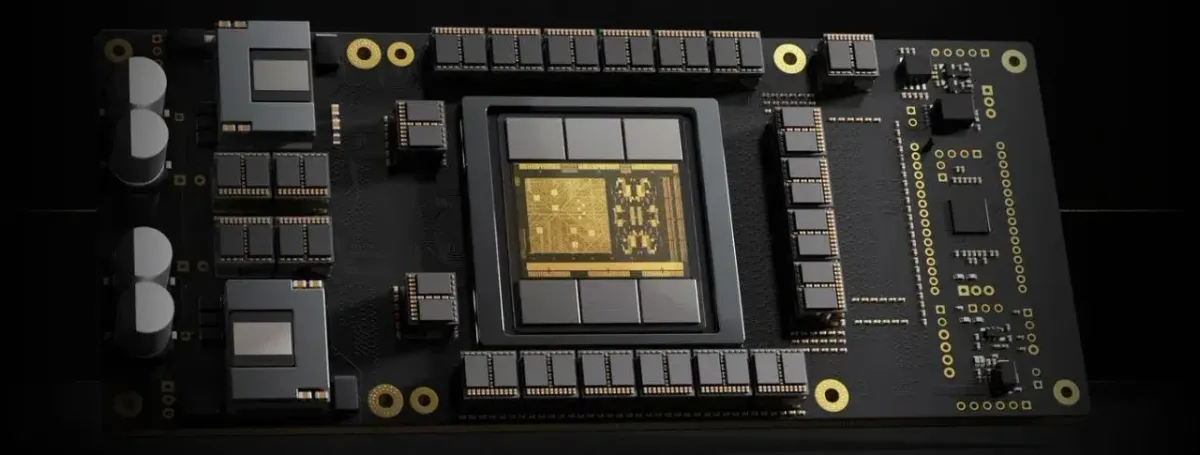
Is it a good time to short NVidia? It's no secret that NVidia is one of the best-performing assets in a bucket of S&P500, dragging the entire market up. So, it would make sense to think about where the price would find its resistance and what could be the catalyst that would initiate stock correction. In the ever-evolving world of artificial intelligence, hardware innovations, i.e. NVidia's Blackwell Platform, serve as critical benchmarks that often dictate the pace of progress across the industry. Recently, a company called "Etched" announced their innovative chip, Sohu ASIC (Application-Specific Integrated Circuit), which, if their claims are true, is an extraordinary achievement in AI computing solutions. I have witnessed numerous disruptive technologies, but the Sohu chip's unique proposition for transformer-based models is particularly interesting since LLMs are so popular today. Should NVidia be worried?
The Sohu ASIC chip represents a significant leap forward in dedicated AI acceleration. Unlike general-purpose AI accelerators like NVidia GPUs, Sohu is engineered to supercharge transformer models. Transformers require massive computational resources due to their reliance on complex tensor (matrix) multiplications. Sohu addresses this by featuring bespoke architecture that optimizes these specific operations, allowing it to deliver performance unrivalled by traditional GPUs. While consuming only 10 watts of power, sohu claims to deliver a 20-fold improvement in both speed and cost efficiency and if you read my previous article about the costs of running NVidia GPUs on-prem, you would know that electricity bill is one of the most crucial considerations for any business.
Another important aspect is model inference latency. Recently, I spoke with a founder of AsiaBots. They have implemented a ChatBot that can talk to a human live in almost real-time. Essentially, it's a system of 3 AI models: Speech to text (ASR) -> Language Model (LLM) -> Text to Speech (TTS), and their biggest challenge was latency because it takes time for each model to complete the inference. Etched guys are claiming that their chip significantly outpaces Nvidia's next-generation Blackwell B200 GPUs, with an output of over 500,000 tokens per second on the Llama 70B model. This translates to exponential advancements in operational efficiency and economic viability.
While the benefits are clear for Transformer-based models, the adoption of Sohu will be limited with other types of models due to its specialized nature. Unlike versatile does-it-all NVidia GPUs, Sohu ASIC is engineered specifically for transformers. This is why it is not possible to use it for other AI models like CNNs (Convolutional Neural Networks) or RNNs (Recurrent neural networks) or LSTMs (Long short-term memory). The rapid evolution of AI architectures requires a cautious approach. Today's leading Transformer architecture could be rendered obsolete by newer innovations in a few years or even months. There might be a new architecture coming out to challenge Transformers dominance, and it's called xLSTM.
The Sohu chip by Etched is a thrilling development that underscores the dynamic nature of AI hardware innovation. For organizations interested to jump on a bandwagon of the next wave of AI transformation, Sohu could potentially offer compelling advantages, particularly for those heavily invested in transformer-based models.